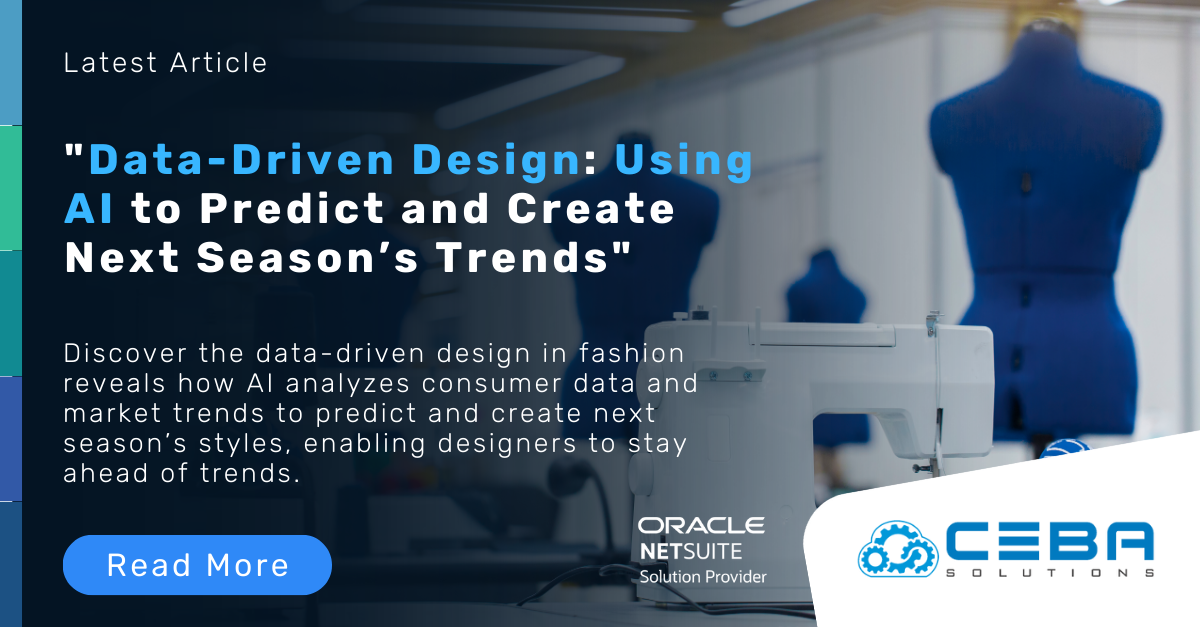
Data-Driven Design: Using AI to Predict and Create Next Season’s Trends
Data-Driven Design: Using AI to Predict and Create Next Season’s Trends
In the ever-evolving world of fashion, staying ahead of trends is crucial for success. Artificial Intelligence (AI) is revolutionizing this aspect by enabling data-driven design approaches that predict and shape future trends. This transformative technology harnesses vast amounts of data to forecast what styles, colors, and materials will resonate with consumers in upcoming seasons. This blog delves into how AI is being utilized to guide the design process, ensuring that fashion brands remain innovative and market-relevant.
1. Trend Prediction Through Big Data Analysis
AI algorithms analyze large datasets collected from a variety of sources including social media, runway shows, current market analytics, and consumer purchasing patterns. This analysis provides a comprehensive view of emerging trends far more quickly and accurately than traditional market research methods.
How It Works:
- Data Collection: AI systems gather data from multiple sources, such as fashion blogs, social media platforms, e-commerce sites, and fashion shows. This includes text, images, and videos that represent current trends and consumer interests.
- Data Processing: Using natural language processing (NLP) and computer vision, AI can interpret the gathered data, categorizing it into different fashion elements like colors, patterns, and styles.
- Trend Analysis: Machine learning algorithms analyze the processed data to identify patterns and predict upcoming trends. This involves statistical analysis and trend scoring to determine the popularity of certain elements.
Benefits:
- Anticipatory Designs: Allows designers to create collections that align with predicted consumer trends, increasing the likelihood of market success. Brands can launch products that are more likely to be in demand, reducing the risk of unsold inventory.
- Responsive Fashion Cycles: Brands can react more swiftly to emerging trends, giving them a competitive edge in a fast-paced industry. This agility allows for quicker design-to-market timelines, keeping brands relevant and trendy.
2. Customer Behavior Insights
AI not only predicts general market trends but also dives deep into individual consumer behavior. By understanding preferences and purchasing habits at a granular level, AI helps designers create personalized and targeted collections that meet specific customer desires.
How It Works:
- Consumer Profiling: AI systems create detailed profiles of customers based on their online activities, purchase history, and social media interactions.
- Preference Prediction: Machine learning models analyze these profiles to predict individual preferences for styles, colors, and brands.
- Personalization: Designers can use these insights to tailor their collections to different customer segments, ensuring that the products resonate on a personal level.
Benefits:
- Enhanced Customer Satisfaction: Products that resonate on a personal level are more likely to satisfy and retain customers. Personalized fashion experiences can lead to higher customer loyalty and repeat purchases.
- Increased Sales Potential: Personalized offerings are typically more compelling to consumers, leading to higher sales volumes. Brands can target specific customer segments with customized marketing campaigns, increasing conversion rates.
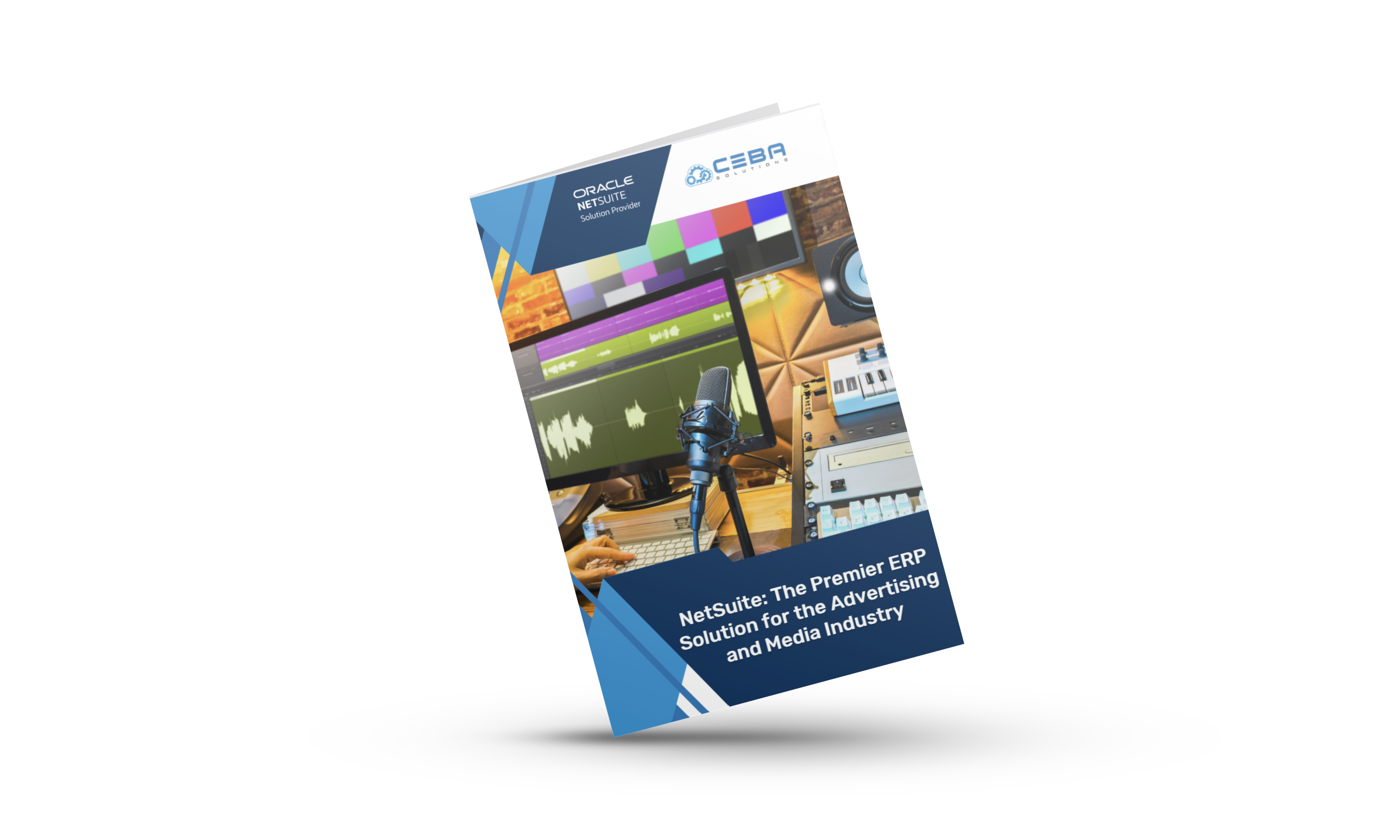
3. Automated Design Elements
AI can automate certain elements of the design process, such as pattern generation and color matching. This technology can suggest design modifications or entirely new concepts based on historical data and emerging trends, assisting designers in exploring creative avenues they might not have considered.
How It Works:
- Pattern Generation: AI algorithms can generate new patterns by analyzing existing designs and combining different elements in innovative ways.
- Color Matching: AI systems can recommend color palettes based on trending colors and historical data, ensuring that the designs are visually appealing and market-relevant.
- Design Suggestions: AI can propose design alterations or new concepts by analyzing current trends and consumer preferences.
Benefits:
- Creative Augmentation: Provides designers with innovative design options, enhancing creativity without replacing the designer’s intuition. This collaboration between AI and human designers can lead to unique and original collections.
- Efficiency Improvements: Speeds up the design process by automating routine tasks, allowing designers to focus on more strategic and creative aspects. This increased efficiency can reduce time-to-market and operational costs.
4. Prototype Testing and Feedback
AI-driven simulations can create and test virtual prototypes before a single piece of fabric is cut. This technology can predict how a design will perform in the market based on virtual feedback, significantly reducing the resources typically required for physical prototyping and testing.
How It Works:
- Virtual Prototyping: AI creates digital prototypes of designs, simulating how they will look and fit on different body types.
- Market Simulation: AI models can simulate market conditions and consumer reactions to the virtual prototypes, providing early feedback on potential design successes or failures.
- Feedback Integration: Designers can use this feedback to make adjustments to the designs before moving to physical production.
Benefits:
- Cost Reduction: Decreases the cost of sample production and physical testing. Virtual prototyping reduces the need for multiple physical samples, saving time and resources.
- Market Feedback Analysis: Provides early insights into potential market reactions, allowing for design tweaks before full-scale production begins. This proactive approach can prevent costly mistakes and enhance product success rates.
5. Sustainability Through Predictive Analytics
By accurately forecasting which designs will be popular, AI helps minimize overproduction, a significant issue in the fashion industry. Predictive analytics ensures that production aligns more closely with market demand, contributing to sustainability efforts.
How It Works:
- Demand Forecasting: AI models predict future demand for different products based on historical sales data and emerging trends.
- Inventory Management: These predictions help in planning production quantities, reducing the risk of overproduction and excess inventory.
- Sustainable Practices: AI can also suggest sustainable materials and production methods, aligning fashion design with environmental goals.
Benefits:
- Waste Reduction: Less overproduction means less waste, making the fashion industry more sustainable. This approach supports environmental conservation and reduces the carbon footprint of fashion brands.
- Resource Optimization: Efficient use of materials and resources protects the environment and reduces manufacturing costs. Sustainable practices can also enhance brand reputation and attract eco-conscious consumers.
Conclusion
AI's role in data-driven design is transforming how fashion brands forecast, create, and market their products. By integrating AI into their design processes, brands can not only anticipate market demands but also cater to individual consumer preferences, all while enhancing creativity and sustainability. As AI technology continues to evolve, its influence on fashion design is expected to grow, shaping the future of the industry in profound ways.
By leveraging AI, fashion brands can stay ahead of trends, personalize their offerings, streamline their design processes, and commit to sustainability. This synergy of technology and creativity is setting the stage for a new era in fashion design, where data-driven insights lead to innovative and market-relevant collections.