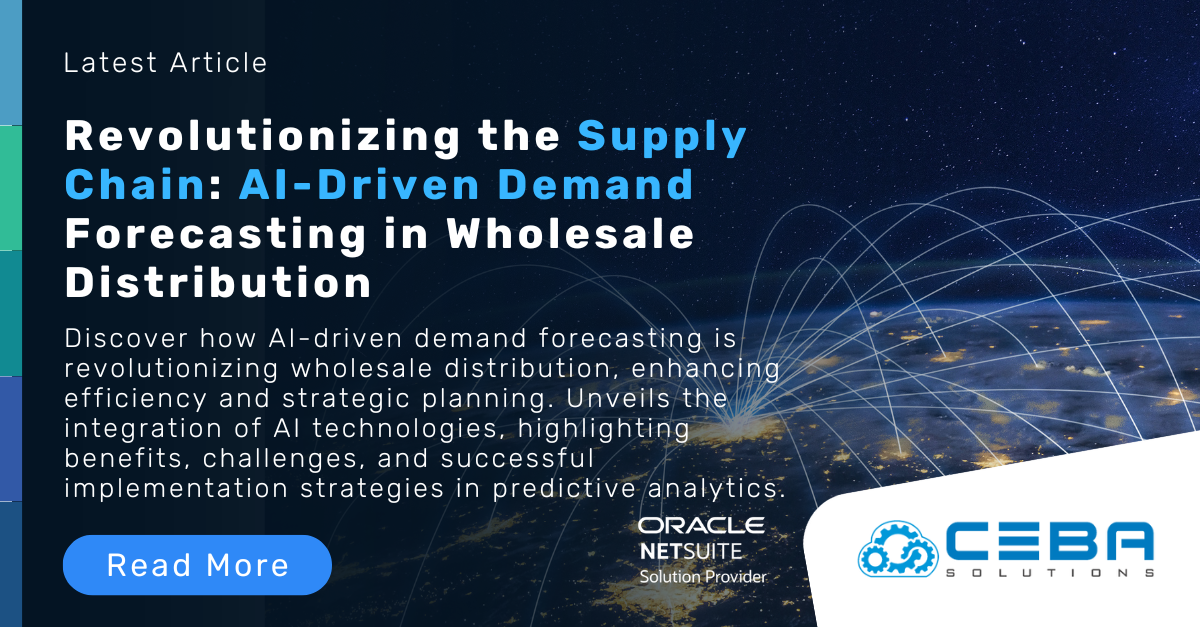
Revolutionizing the Supply Chain: AI-Driven Demand Forecasting in Wholesale Distribution
Revolutionizing the Supply Chain: AI-Driven Demand Forecasting in Wholesale Distribution
In the ever-evolving landscape of wholesale distribution, efficient supply chain management is crucial for maintaining competitiveness and profitability. One of the most significant challenges wholesalers face is accurately predicting customer demand to optimize inventory levels, reduce costs, and enhance service levels. Traditional demand forecasting methods, often relying on historical data and manual adjustments, can fall short in addressing the complexities of modern markets. Enter artificial intelligence (AI)-driven demand forecasting—a transformative approach that leverages advanced algorithms and machine learning to revolutionize supply chain management. This article explores how AI-driven demand forecasting is transforming wholesale distribution, its benefits, and practical implementation strategies.
The Importance of Demand Forecasting in Wholesale Distribution
Accurate demand forecasting is essential for several reasons:
- Inventory Optimization: Ensuring that the right amount of stock is available to meet customer demand without overstocking.
- Cost Reduction: Minimizing costs associated with excess inventory, stockouts, and expedited shipping.
- Customer Satisfaction: Providing reliable product availability, leading to higher customer satisfaction and loyalty.
- Operational Efficiency: Streamlining procurement, production, and distribution processes to enhance overall operational efficiency.
How AI-Driven Demand Forecasting Works
AI-driven demand forecasting utilizes machine learning algorithms to analyze vast amounts of data, identify patterns, and make accurate predictions about future demand. Here’s how it works:
- Data Collection and Integration
- Comprehensive Data Gathering: Collect data from various sources, including historical sales, market trends, economic indicators, seasonal patterns, and external factors like weather and social media trends.
- Data Integration: Integrate data from different systems, such as enterprise resource planning (ERP), customer relationship management (CRM), and point-of-sale (POS) systems, to create a unified dataset for analysis.
- Machine Learning Algorithms
- Pattern Recognition: Machine learning algorithms analyze historical data to identify patterns and correlations that influence demand.
- Predictive Modeling: Algorithms such as regression analysis, time series forecasting, and neural networks create predictive models that forecast future demand based on identified patterns and trends.
- Real-Time Adjustments
- Dynamic Forecasting: AI models continuously update forecasts in real-time as new data becomes available, allowing for dynamic adjustments to changing market conditions and customer behaviors.
- Anomaly Detection: AI can detect anomalies and outliers in data, enabling quick responses to unexpected events or demand spikes.
Benefits of AI-Driven Demand Forecasting
- Improved Accuracy
- Data-Driven Insights: AI-driven forecasting relies on comprehensive data analysis, reducing the reliance on human intuition and manual adjustments.
- Enhanced Precision: Advanced algorithms consider a multitude of factors and complex relationships, leading to more accurate predictions.
- Cost Savings
- Inventory Reduction: Accurate demand forecasting helps reduce excess inventory, lowering holding costs and minimizing waste.
- Efficient Resource Allocation: Optimizing inventory levels and procurement schedules reduces costs associated with stockouts, overstocking, and emergency orders.
- Enhanced Customer Satisfaction
- Reliable Availability: Ensuring that products are available when customers need them enhances satisfaction and loyalty.
- Reduced Stockouts: Minimizing stockouts prevents lost sales and maintains customer trust.
- Operational Efficiency
- Streamlined Processes: Accurate forecasts enable better planning and coordination across procurement, production, and distribution, enhancing overall efficiency.
- Proactive Management: AI-driven insights allow for proactive management of supply chain disruptions and demand fluctuations.
Implementing AI-Driven Demand Forecasting
- Data Preparation
- Data Quality: Ensure data accuracy, completeness, and consistency. Clean and preprocess data to handle missing values, outliers, and inconsistencies.
- Unified Dataset: Integrate data from various sources to create a comprehensive and unified dataset for analysis.
- Algorithm Selection
- Choose Appropriate Models: Select machine learning algorithms based on specific forecasting needs and data characteristics. Common models include linear regression, ARIMA (AutoRegressive Integrated Moving Average), and deep learning models like LSTM (Long Short-Term Memory) networks.
- Model Training and Validation: Train models using historical data and validate their accuracy through testing and refinement.
- System Integration
- Seamless Integration: Integrate AI-driven forecasting models with existing ERP, CRM, and inventory management systems to ensure seamless data flow and real-time updates.
- User-Friendly Interface: Develop user-friendly interfaces that allow business users to interact with forecasting models and gain actionable insights.
- Continuous Monitoring and Improvement
- Performance Tracking: Monitor the performance of AI-driven forecasting models using key performance indicators (KPIs) such as forecast accuracy, inventory turnover, and customer satisfaction.
- Model Refinement: Continuously update and refine models with new data and feedback to maintain accuracy and relevance.
- Change Management and Training
- Stakeholder Engagement: Secure buy-in from key stakeholders, including management, supply chain teams, and IT, to ensure successful implementation and adoption of AI-driven forecasting.
- Training Programs: Provide training for staff to understand and effectively use AI tools and insights, emphasizing the benefits of data-driven decision-making.
Future Trends in AI-Driven Demand Forecasting
- Integration with IoT and Big Data
- IoT Data Utilization: Leveraging data from IoT devices, such as smart sensors and connected products, provides real-time insights into inventory levels, product usage, and supply chain conditions.
- Big Data Analytics: Advanced big data analytics techniques enhance the ability to process and analyze massive datasets, leading to more accurate and granular demand forecasts.
- AI-Powered Supply Chain Platforms
- End-to-End Solutions: AI-powered supply chain platforms offer end-to-end visibility and optimization, integrating demand forecasting with procurement, production, and distribution planning.
- Collaborative Networks: AI enables collaborative forecasting and planning across the supply chain, fostering greater coordination and efficiency among suppliers, manufacturers, and distributors.
- Sustainability and Ethical AI
- Sustainable Practices: AI-driven demand forecasting supports sustainable supply chain practices by optimizing inventory levels, reducing waste, and minimizing carbon footprints.
- Ethical Considerations: Ensuring ethical AI practices, such as transparency, fairness, and data privacy, is crucial for building trust and maintaining compliance with regulations.
Conclusion
AI-driven demand forecasting is revolutionizing the wholesale distribution industry by providing accurate, data-driven insights that enhance supply chain management. By leveraging advanced machine learning algorithms and real-time data analysis, businesses can optimize inventory levels, reduce costs, and improve customer satisfaction. Implementing AI-driven forecasting requires careful data preparation, algorithm selection, system integration, and continuous improvement, but the benefits far outweigh the challenges. As technology continues to evolve, AI-driven demand forecasting will play an increasingly vital role in navigating the complexities of modern supply chains and driving long-term success in the wholesale distribution industry.