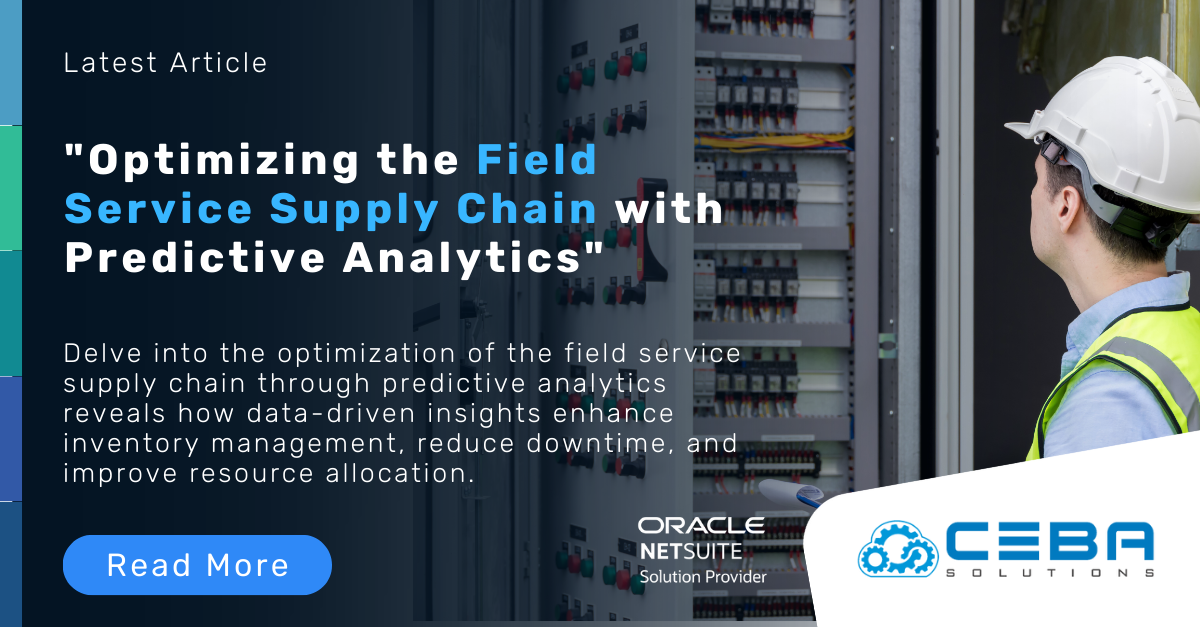
Optimizing the Field Service Supply Chain with Predictive Analytics
Optimizing the Field Service Supply Chain with Predictive Analytics
Predictive analytics is revolutionizing the field service supply chain, transforming traditional reactive operations into proactive and strategic management systems. This powerful tool enables businesses to forecast future demands, optimize inventory levels, and enhance overall operational efficiency by using data-driven insights. This article explores how predictive analytics is being applied within the field service sector to streamline processes, reduce costs, and improve service delivery.
1. Predicting Equipment Failures
Predictive analytics uses historical data and machine learning algorithms to predict when equipment is likely to fail, allowing service providers to intervene before an actual breakdown occurs. This approach significantly reduces downtime and avoids the costs and disruptions associated with unexpected equipment failures.
Benefits:
- Reduced Downtime: Proactively repairing or replacing parts before they fail keeps systems running smoothly and prevents costly interruptions. This predictive maintenance approach ensures continuous operation and maximizes uptime.
- Enhanced Customer Satisfaction: Minimizing equipment downtime improves reliability and service, enhancing customer satisfaction and loyalty. Customers appreciate the proactive service that prevents inconvenient breakdowns.
Real-World Application:
Companies like Siemens use predictive analytics to monitor and maintain their industrial equipment. By analyzing historical and real-time data, they can predict potential failures and perform maintenance before issues escalate, ensuring uninterrupted operation for their clients.
2. Inventory Optimization
Managing inventory efficiently is critical in field service operations to ensure that the right parts are available at the right time. Predictive analytics helps companies anticipate future demand based on trends, seasonal fluctuations, and other factors, enabling them to optimize their stock levels and reduce carrying costs.
Benefits:
- Decreased Inventory Costs: Maintaining optimal inventory levels reduces excess stock and associated costs. Predictive analytics helps in minimizing waste and avoiding overstock situations, thereby reducing holding costs.
- Improved Service Levels: Ensures the availability of necessary parts when and where they are needed, thus avoiding delays in service delivery. Timely access to the right parts enhances the ability to meet service commitments efficiently.
Real-World Application:
Retail giants like Amazon use predictive analytics to manage their vast inventory. This technology allows them to forecast demand accurately and maintain optimal stock levels, ensuring that products are available to customers while minimizing excess inventory.
3. Efficient Resource Allocation
Predictive analytics also assists in the efficient allocation of resources, including technicians and transportation. By predicting service demands, companies can better plan their workforce distribution, ensuring that technicians with the right skills are available to meet service demands efficiently.
Benefits:
- Increased Operational Efficiency: Smarter allocation of resources reduces wasted efforts and costs. Predictive analytics ensures that resources are utilized effectively, improving productivity and reducing idle time.
- Enhanced Response Times: Faster and more accurate service responses improve overall customer service quality. Efficient resource allocation ensures that technicians can respond quickly to service calls, enhancing customer satisfaction.
Real-World Application:
Telecom companies like AT&T use predictive analytics to allocate field technicians efficiently. By analyzing service requests and predicting demand, they can dispatch the right technicians to the right locations, ensuring timely and effective service delivery.
4. Maintenance Scheduling
Using predictive analytics to schedule maintenance activities can significantly enhance service efficiency. By analyzing data on equipment usage and performance, businesses can schedule maintenance only when needed, rather than adhering to a less efficient cyclical schedule.
Benefits:
- Extended Equipment Lifespan: Regular, data-driven maintenance extends the operational life of equipment. Predictive scheduling ensures that maintenance is performed at optimal times, preventing wear and tear and prolonging equipment durability.
- Cost Savings: Preventative maintenance is less expensive than major repairs, resulting in significant cost savings over time. Proactive maintenance reduces the likelihood of costly emergency repairs and replacements.
Real-World Application:
General Electric (GE) uses predictive analytics to schedule maintenance for their aircraft engines. By predicting when maintenance is needed based on usage patterns and performance data, GE can perform timely maintenance, reducing the risk of in-flight failures and costly repairs.
5. Demand Forecasting
Predictive analytics enables businesses to forecast demand for field services, which is crucial for planning and scalability. This forecasting helps manage the workforce and inventory in anticipation of increased service needs, ensuring that the business can scale operations up or down as required.
Benefits:
- Better Planning: Accurate demand forecasts allow for better strategic planning and resource allocation. Companies can plan their operations more effectively, ensuring that they are prepared to meet customer needs.
- Scalability: Ensures that operations can be scaled to meet demand without sacrificing service quality. Predictive analytics provides insights that help businesses adjust their operations to match demand fluctuations, maintaining high service standards.
Real-World Application:
Utility companies like National Grid use predictive analytics to forecast demand for electricity and gas services. By analyzing historical data and usage patterns, they can predict peak demand periods and ensure that they have sufficient resources to meet customer needs.
Conclusion
Predictive analytics is a game-changer for optimizing the field service supply chain. By enabling companies to anticipate future needs and respond proactively, predictive analytics not only enhances operational efficiencies but also drives cost savings and improves customer satisfaction. As technology continues to advance, leveraging predictive analytics will become increasingly critical for field service providers seeking to maintain competitive advantage and meet evolving customer expectations.
The integration of predictive analytics into field service operations represents a significant advancement in how services are delivered. Companies that adopt these technologies can expect to see improvements in efficiency, cost management, and customer satisfaction, positioning themselves as leaders in the industry. As predictive analytics continues to evolve, its role in transforming the field service supply chain will only grow, offering even greater opportunities for innovation and improvement.