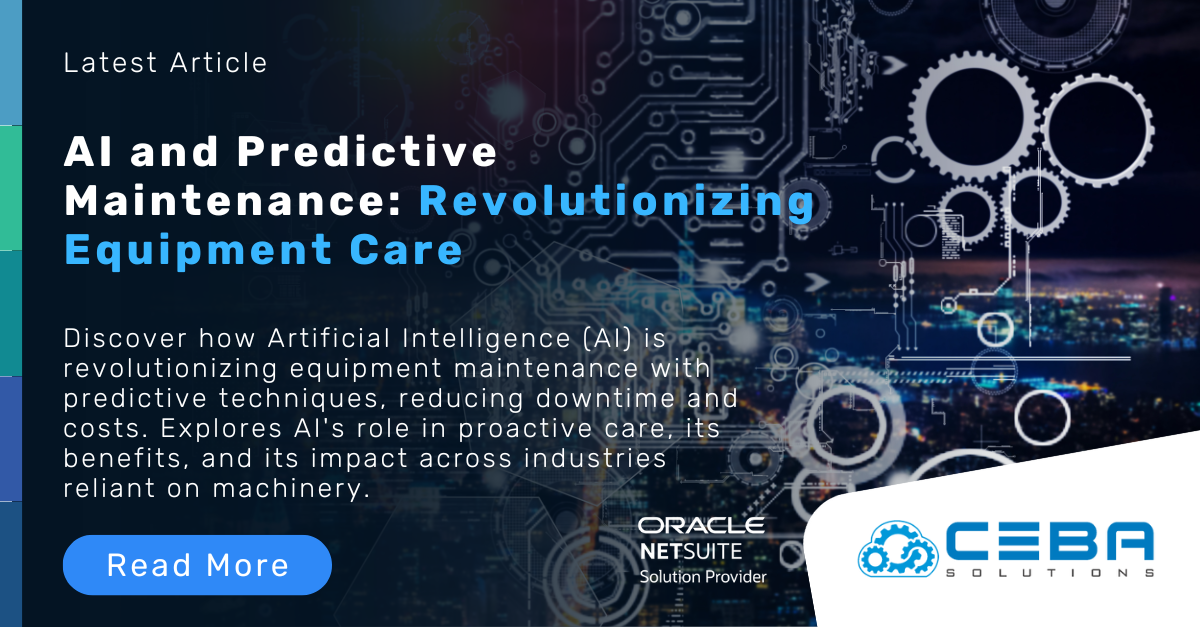
AI and Predictive Maintenance: Revolutionizing Equipment Care
AI and Predictive Maintenance: Revolutionizing Equipment Care
In today's industrial and manufacturing landscapes, the maintenance of equipment is a critical aspect of ensuring operational efficiency, minimizing downtime, and reducing costs. Traditional maintenance strategies, such as reactive and preventive maintenance, often fall short in optimizing equipment care and performance. However, the advent of artificial intelligence (AI) and predictive maintenance is revolutionizing how businesses manage their equipment. By leveraging AI, companies can predict equipment failures before they occur, enabling proactive maintenance that enhances reliability and efficiency. This article explores how AI-driven predictive maintenance is transforming equipment care and the benefits it brings to various industries.
Understanding Predictive Maintenance
Predictive maintenance (PdM) is a proactive approach that uses data analysis tools and techniques to detect anomalies and predict equipment failures. Unlike preventive maintenance, which follows a scheduled timetable, predictive maintenance relies on the actual condition of the equipment. AI enhances PdM by analyzing vast amounts of data from sensors and other sources to predict when maintenance should be performed. This approach ensures maintenance is only conducted when necessary, preventing unexpected failures and extending the lifespan of equipment.
The Role of AI in Predictive Maintenance
AI plays a crucial role in predictive maintenance by utilizing machine learning algorithms, data analytics, and real-time monitoring to provide actionable insights. Here are key components of AI-driven predictive maintenance:
- Data Collection and Integration
- Sensors and IoT Devices: AI-driven PdM systems rely on data collected from various sensors and Internet of Things (IoT) devices installed on equipment. These sensors monitor parameters such as temperature, vibration, pressure, and humidity in real-time.
- Data Integration: Integrating data from different sources, including historical maintenance records, operational data, and environmental conditions, provides a comprehensive view of equipment health.
- Machine Learning Algorithms
- Anomaly Detection: Machine learning algorithms analyze data to detect anomalies and deviations from normal operating conditions. These algorithms can identify patterns that indicate potential failures.
- Predictive Modeling: AI models are trained on historical data to predict future equipment failures. These models continuously learn and improve their accuracy over time.
- Real-Time Monitoring and Analysis
- Continuous Monitoring: AI systems continuously monitor equipment in real-time, providing up-to-date insights into its condition. This allows for immediate detection of issues as they arise.
- Advanced Analytics: AI-driven analytics tools process and analyze data to provide actionable insights. These tools can identify root causes of issues, predict failure timelines, and recommend maintenance actions.
Benefits of AI-Driven Predictive Maintenance
- Reduced Downtime and Increased Reliability
- Proactive Maintenance: By predicting equipment failures before they occur, AI-driven PdM allows for proactive maintenance. This reduces unplanned downtime and ensures equipment operates reliably.
- Extended Equipment Lifespan: Timely maintenance based on actual equipment conditions helps extend the lifespan of assets, reducing the need for costly replacements.
- Cost Savings
- Optimized Maintenance Schedules: Predictive maintenance ensures that maintenance activities are performed only when necessary, reducing unnecessary maintenance costs.
- Lower Repair Costs: Early detection of issues allows for minor repairs before they escalate into major failures, reducing repair costs.
- Enhanced Safety and Compliance
- Improved Safety: Predictive maintenance helps identify potential safety hazards before they lead to accidents, enhancing workplace safety.
- Regulatory Compliance: AI-driven PdM systems can help ensure compliance with industry regulations and standards by maintaining equipment in optimal condition.
- Data-Driven Decision Making
- Actionable Insights: AI provides actionable insights that enable data-driven decision-making. Maintenance teams can prioritize tasks, allocate resources effectively, and make informed decisions.
- Performance Optimization: Continuous monitoring and analysis of equipment performance help identify areas for optimization, improving overall operational efficiency.
Implementing AI-Driven Predictive Maintenance
- Assessing Readiness and Setting Objectives
- Readiness Assessment: Evaluate the current state of your equipment, data infrastructure, and maintenance processes. Assess readiness for implementing AI-driven PdM.
- Clear Objectives: Define clear objectives for the predictive maintenance program, such as reducing downtime, lowering maintenance costs, and improving equipment reliability.
- Deploying Sensors and IoT Devices
- Sensor Installation: Install sensors and IoT devices on critical equipment to collect real-time data. Ensure sensors are calibrated and provide accurate readings.
- Data Connectivity: Establish robust data connectivity to ensure seamless data transfer from sensors to the AI system.
- Building and Training AI Models
- Data Collection: Collect historical and real-time data to train AI models. Ensure data quality and completeness.
- Model Training: Use machine learning algorithms to train predictive models. Continuously update and refine models with new data to improve accuracy.
- Integrating with Existing Systems
- System Integration: Integrate the AI-driven PdM system with existing maintenance management systems, enterprise resource planning (ERP) systems, and other relevant platforms.
- User Interface: Develop user-friendly interfaces that provide maintenance teams with easy access to insights and recommendations.
- Training and Change Management
- Employee Training: Train maintenance personnel on using AI-driven PdM tools and interpreting the insights provided. Emphasize the importance of data-driven decision-making.
- Change Management: Implement change management strategies to ensure smooth adoption of the new PdM system. Address any resistance and highlight the benefits.
- Continuous Improvement and Monitoring
- Performance Metrics: Establish key performance indicators (KPIs) to monitor the effectiveness of the predictive maintenance program. Common KPIs include mean time between failures (MTBF), maintenance costs, and downtime reduction.
- Regular Reviews: Conduct regular reviews of the PdM system’s performance and make necessary adjustments. Continuously seek opportunities for improvement.
Challenges and Considerations
- Data Quality and Management
- Data Accuracy: Ensure that the data collected is accurate, reliable, and representative of actual equipment conditions.
- Data Volume: Manage the large volumes of data generated by sensors and IoT devices. Implement data storage and processing solutions that can handle big data.
- Initial Costs and ROI
- Upfront Investment: Be prepared for the initial costs associated with implementing AI-driven PdM, including sensors, data infrastructure, and AI tools.
- Long-Term ROI: Focus on the long-term return on
investment, considering the cost savings, reduced downtime, and increased equipment lifespan that AI-driven predictive maintenance can deliver.
- Integration and Interoperability
- Seamless Integration: Ensure that the AI-driven PdM system integrates seamlessly with existing maintenance and operational systems. Address any interoperability issues to avoid data silos and ensure smooth data flow.
- Vendor Collaboration: Work closely with technology vendors to customize AI solutions that meet your specific needs and address industry-specific challenges.
- Security and Privacy
- Data Security: Implement robust cybersecurity measures to protect sensitive data collected from equipment and sensors. Ensure that data is encrypted and access is controlled.
- Privacy Compliance: Ensure compliance with data privacy regulations and standards. Address any concerns related to data collection and usage.
Conclusion
AI-driven predictive maintenance is revolutionizing equipment care by providing advanced solutions that enhance reliability, reduce costs, and improve operational efficiency. By leveraging machine learning algorithms, real-time data analysis, and predictive modeling, businesses can transition from reactive and preventive maintenance strategies to proactive maintenance. Implementing AI-driven PdM requires careful planning, data integration, and employee training, but the benefits far outweigh the challenges. As AI technology continues to evolve, its impact on equipment maintenance will only grow, driving innovation and excellence across various industries. Embracing AI for predictive maintenance is not just a competitive advantage but a necessary step toward achieving long-term operational success and sustainability.